Every year, Intento releases a comprehensive breakdown of the current state of the Machine Translation market for MT newbies and seasoned veterans alike.
This is the sixth year we have run this report, dating back to 2017. In it, we evaluate all commercially available systems that our tech recognizes throughout the market on the best data we can gather. The 2022 report was a collaborative effort with e2f, providing a top-notch data set. We translated about 1M words to prepare, focusing on 11 language pairs and 9 different domains.
At AMTA 2022 in Orlando, Intento CEO Konstantin Savenkov and e2f CEO Michel Lopez broke down the data selection and preparation process, the report methodology, principal scores to rely on when studying MT outcomes, and the main report outcomes (best performing MT engines for every language/domain combination). If you missed his lecture, keep reading for a full summary.
• • •
“We feel that this data is something every MT user should have access to.” (Konstantin Savenkov, Co-founder and CEO, Intento)
• • •
The Machine Translation Landscape
Looking at the MT landscape in 2022, we have 54 vendors providing models. Nowadays, most of these models are neural, so we classify them based on how you can utilize the MT. Many vendors provide generic stock models, while others are pre-trained for specific verticles such as Pharma or E-commerce. At the same time, others are providing custom terminology support, allowing you to add glossaries without training the model. ‘Automatic domain adaptation’ lets you press a button to customize your model using Translation Memory (TM). Sometimes, you need to provide this data to a team of linguists, which we call ‘manual domain adaptation.’
Next year, we expect to split Auto Domain Adaptation into ‘static adaptation’ (batch training on TM) and ‘dynamic adaptation,’ where you can train your model on every sentence.
Our State of the MT 2022 report was done on stock pre-trained models, meaning that they are uncustomized. Customization allows you to improve your model based on your own data, which could potentially change the overall outcome and rankings.
• • •
Datasets and Preparation
We initially began running these reports on WMT data, used by all MT vendors to train and evaluate their models. About a year ago, we switched to TAUS data — but we still had a problem as many MT providers purchase their data from TAUS, creating an unfair advantage for them.
This year, we wanted to ensure that the data was high quality and that nobody would have access to the relevant translations before the report.
We have collected English texts from various public sources, making sure there’s no translation for them available online. The source texts were translated by Subject Matter Experts at e2f, with our experts stating that this was the best dataset they had ever seen.
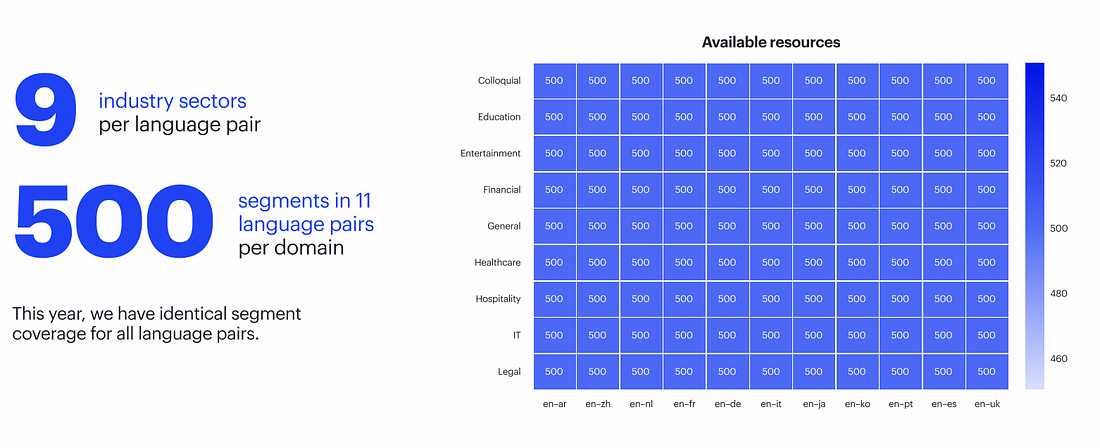
Evaluation Approach
Selecting the best model with the highest score makes less sense than expected. There may be other models with a slightly lower score yet similar quality.
Our goal was to identify the top-ranking models in the same confidence interval as the best model. We also made the same retrospective analysis of the commercial evaluation projects (which invariably involve human evaluation) and found that in about 95% of cases, if the model got to this top band from the reference human translation, it should be a winner.
You’ll notice that instead of 95%-confidence intervals, we use 83% — this is because when you compare means of distributions, this is equivalent to 95%-confidence intervals for the distributions themselves (see the report for scientific references).
• • •
Which Score to Use
After calculating all the semantic and syntactic scores available and their correlations, we decided to go with COMET (semantic) for this year’s report. We found that COMET has the most correlation with reviewer judgment.
COMET predicts MT quality using information from both the source input and the reference translation. It may penalize certain nuances in translations, such as a different tone of voice or gender, due to the fact this metric was trained on a specific dataset and inherited its biases.
• • •
Best MT Engines per Industry Sector
You can read about all of our findings — and examine the full summary table — in the official 2022 report, which is available to download for free here.
Among the insights you’ll find inside, you’ll see:
- The 16 engines that are among the most statistically significant leaders for 9 domains and 11 language pairs
- The languages and language pairs where many engines perform best.
- The 3 domains require a careful choice of MT vendor, as relatively few perform in the top tier.
- The 2 domains where customization may carry more weight.
Another finding is that language support has grown significantly, primarily thanks to NiuTrans, Meta, and Alibaba. Across all models, there are now 125k language pairs supported.
We’ve also seen that the number of vendors is consistently rising, and this year we have 5 new vendors, resulting in a total of 54 MT vendors. Among these vendors is an open-source model from Meta, ranking among the top choices for Spanish.
• • •
Download the full report to get the inside scoop on the bleeding edge of MT innovation. For any further questions or a free consultation, book a meeting on our demo page.