Successfully applying MT to grow your business is not always a successful venture. This may not be due to the MT engines or the nature of your operation, but rather you just need the right methodology and tools to make MT work for you. That’s why at Intento, we’ve developed an AI-driven toolbox to make sure you can select the right MT for your organization. Intento MT Studio offers meaningful insights in an easy-to-understand manner for a more straightforward and effective way of deploying the best-fit MT.
Here is how your business can benefit from MT Studio:
- Energize your localization efforts without putting more stress on your team.
- Save time and money on the post-editing of the project with well-chosen MT.
- Have your custom model training tailored to the specific context of your project.
Intento users bring their human editing time down by an average of 70%, their integration and AI fees by 80%, and repeatable translation fees by 88%. At the same time, clients see their performance soar to 97% end-user acceptance rates.
This post will cover the entire Intento MT Studio system, from preparing your data and neural models to evaluating this data to discover the best-fit MT, as well as fine-tuning MT output for the most useful results. Keep reading to learn how to improve your MT experience with the Intento MT Studio.
• • •
Common Issues in Data Cleaning and MT Evaluation
If you go through the process without measuring interim results, then you end up with more MT output issues at the end that are actually avoidable by changing your review process. Each of these issues will be a cost to your company, whether that be financially or in your team’s valuable time. By gathering these problems along the way, without interim reviews, you cause the cost to gradually increase as you go.
Training models on messy data
It is common to train models based on all available data, meaning they inevitably end up being trained on messy data. By cleaning your training data, you can avoid inconsistencies in translation or disruptive punctuation like bullet points. This sort of ‘cluttered’ data can undermine your entire project, making custom models vulnerable to further errors. Essentially, if your references aren’t adequate, your MT won’t be either.
Sampling from random segments
The sampling stage is complicated by random sampling. The issue here is that you’re blindly choosing a few segments out of thousands for LQA sampling. There is no reason these samples would lead to meaningful results, and this ‘random sampling’ can actually lead to large groups of data, with relevant feedback for your dataset being missed entirely. As a result, you may miss crucial issues in the evaluation by not paying attention to the little details.
The intelligent way to sample would be to extract the most meaningful segments using different MT evaluation metrics. For example, the segments in which the MT model is most different from all of the others. You can use different metrics and methods to get different slices of data for a more reliable human review. Errors related to LQA human review / random sampling lead to different results from the same data set, and the accuracy of this testing is unpredictable.
Reporting issues too late
A post-editing machine translation (PEMT) report is a common option; however, it only reports issues when it is already too late. You only receive a PEMT report once a user has applied MT for their project and then measure the quality of the selected engine. By the time this information is available, your model is already in production, and you have already spent time and money fixing MT issues. You need a more active evaluation process that detects these errors immediately so that you can fix them along the way.
All of these simple mistakes end up unnecessarily costing both human and financial resources when post-editing or using real-time MT. Intento helps you avoid these costly mistakes, catching and correcting issues as you go for the most efficient and effective MT process. More influence in your MT means that you have more control over your resources to grow a productive business instead of wasting time and money on unnecessary problems.
• • •
Solutions Provided by Intento MT Studio
Preparing your data the right way
Intento provides solutions to these goals through several useful tools so you can actively prepare your data and neural models.
Data Cleaner guarantees useful data, removing all elements from your data that may lead to skewed results later in the process. We do not move forward until we know the data being used will render the best results for each specific project, and only then we implement MT Training for the training of custom models.
MT Customization Analysis checks the quality of new models (after the custom model training) using human reference translation as a baseline. Critically, we are not only using surface-level scores but go deep into the cracks that more traditional methods pass over. We look at the data at the segment level, finding the segments most appropriate for the parameters of the analysis. Going to this level of analysis provides key insights on the issues surrounding the custom models, which are then delivered in an easy-to-understand manner.
The best evaluation practices
The evaluation process has its own set of tools to make sure you are able to deploy the best-fit model for your project.
Scoring selects the top-runner models for evaluation and is one of the most influential steps in decreasing the amount of time and money spent on human evaluation — but do not stop here! Don’t settle on blindly following the corpus-level scores. Dive a bit deeper into the top-performing models and see who is the best fit.
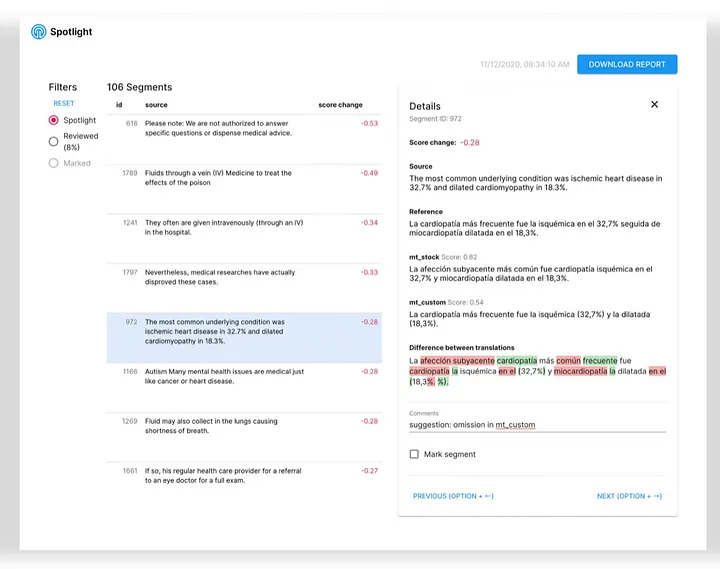
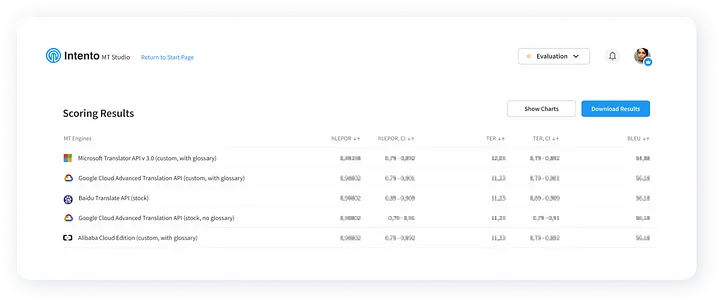
LQA tools conduct a second-level evaluation within this group. A deeper analysis allows us to take a much more thorough look at segment-level performance so that we don’t miss anything important. This level of analysis promises a more accurate depiction of which model is the best-fit solution.
Also, LQA tools utilize smart-sampling techniques to extract valuable segments for human review instead of simply selecting random segments. As discussed above, random sampling is a naive practice that could potentially make your entire operation unproductive. Random sampling also adds too much time to the human review process. By fine-tuning the process and only picking the segments that matter for your particular evaluation, you exponentially cut down the time that is needed for human review and create massive saving opportunities.
Finally, once the best-fit MT is uncovered through Analysis tools, Routing Designer helps you successfully deploy your selection straight away.
Empowering your custom models
You can increase the value of your MT output with custom glossaries and feedback management options.
Glossary Management improves your translations using custom terminology. Glossaries make sure that your organization’s specific terminology, product names, and other cast-specific words are reliably translated the way you want them, no matter the context. These terms will be recognized in any material you are trying to translate and then translated based on your specifications. What really sets Intento Glossaries apart from other vendors is the Intento Abbreviations Glossary. This is a new glossary type expressly developed for ad hoc abbreviations, which works great in e-commerce and customer service cases.
Feedback Management leverages past experiences in MT, based on user recommendations, and provides a tool for easy adjustment to avoid earlier mistakes and replicate effective strategies.
• • •
Cut down evaluation time to hours
Intento MT Studio implements a system specifically designed to save your business’s resources through a more efficient and effective MT process.
An MT evaluation step that would typically take one full day’s worth of analysis through the traditional process will take only 0.5 hours using the Intento smart sampling method. What could take two weeks’ worth of segment review will only take two hours to process.
Don’t waste any more time. Improve your MT evaluation for expert-level translations with Intento’s game-changing tools.